Edge AI technology, also known as the intelligent edge, is a paradigm shift in the realm of artificial intelligence (AI), particularly in deep learning models and machine learning models. This shift is seen in smart devices utilizing cloud computing. Deep learning models and machine learning models have evolved over time, with their roots traced back to the inception of AI itself. This intelligent edge has been further honed by an inference engine. Unlike Cloud AI, Edge AI, with its inference engine and deep learning capabilities, performs data analysis at the source of data generation. By utilizing a machine learning model for these computing tasks, it reduces latency and ensures real-time processing. In today's digital era, edge AI applications, with its cloud computing capabilities and camera technology, holds immense importance due to its ability to run deep learning algorithms on edge devices for computer vision and video analytics.
An Edge AI system, using computer vision, comprises key components such as an inference engine and specialised hardware for training and executing AI models in real time. This system can run efficiently with rapid data transfer. This enables a wide range of applications to run in real time at the intelligent network edge across various industries. From video analysis in surveillance systems to real-time decision-making in autonomous vehicles, Edge AI is revolutionizing how we perceive and implement AI. As we near the end of traditional methods, the run towards more patient, intelligent systems is evident. As we near the end of traditional methods, the run towards more patient, intelligent systems is evident.

Working Mechanism of Edge AI
Data Processing in Edge AI
Edge AI processes real-time data from end patients by applying machine learning algorithms and neural networks at the edge level. This decentralized approach at the network edge reduces latency and bandwidth usage in real time, as data does not need to travel back to a central server for processing. This intelligent edge method ensures an efficient end to data transmission. Instead, edge devices such as IoT sensors can process data locally in real time, utilizing ai hardware to run ai models. For example:
- An autonomous vehicle equipped with Edge AI can quickly process sensor data to make real-time decisions without needing to communicate with a remote server.
- In healthcare, wearable devices using Edge AI can monitor patient vitals and alert medical professionals if abnormal patterns are detected.
This local processing capability is made possible through advanced AI algorithms that enable devices to learn and adapt based on the data they encounter, especially in quantum computing.
Role of IoT Devices in Edge AI
IoT devices play a crucial role in the functioning of Edge AI systems. These devices collect vast amounts of data from their environment, which are then processed locally using sophisticated neural network models. This enables fast decision-making without the need for constant communication with central servers.
For instance, smart home security cameras use Edge AI to detect unusual activity and send alerts directly to homeowners' smartphones. Similarly, industrial IoT sensors leverage edge computing capabilities for real-time equipment monitoring and predictive maintenance.
The integration between IoT devices and Edge AI offers significant benefits including increased efficiency, improved privacy protection, reduced network congestion, among others.
Decision Making Process in Edge AI Systems
Edge AI systems make decisions based on the insights derived from analyzing local data using machine learning algorithms. The decision-making process typically involves these steps:
- Data collection: IoT devices gather raw data from their surroundings.
- Preprocessing: The collected data is cleaned and formatted for analysis.
- Analysis: Machine learning models analyze the preprocessed data to extract meaningful insights.
- Decision making: Based on these insights, the system makes informed decisions or triggers specific actions.
For example, an agricultural drone equipped with multispectral sensors might use edge computing capabilities to analyze crop health in real-time during flight and adjust its spraying pattern accordingly.
Real-Time Analytics in Edge Computing
Real-time analytics is a key element of the working mechanism of edge computing. By processing data at its source (i.e., on the device itself), edge computing enables real-time analytics that would be impossible if relying solely on traditional cloud-based solutions due to latency issues.
Consider a factory floor populated by several robotic arms controlled by an edge-enabled system; it could continually monitor their operation status through embedded sensors and instantly halt any malfunctioning arm before it causes damage or injury.
Application of Machine Learning Algorithms at The Edge Level
Machine learning algorithms are applied at the edge level primarily for two reasons – speed and privacy:
- Speed: By running ML models locally on edge devices like smartphones or drones instead of sending all collected information back to a central server for analysis (which takes time), response times are drastically improved.
- Privacy: Sensitive information remains within local hardware rather than being sent over potentially insecure networks where it could be intercepted or compromised.
Common applications include facial recognition technology used in security systems or voice recognition software integrated into smart home assistants like Amazon's Alexa or Google Home.
Edge AI Applications Benefits and Use Cases
Advantages of Edge Computing
Edge computing brings to the table a multitude of benefits, particularly for edge ai applications. Reduced latency is one such advantage. With data processing happening closer to the source on edge devices, there's less time delay in transmitting information across the network edge. This low latency can be critical in situations where real-time decision making is required.
The privacy aspect also gets a significant boost with edge computing. Since data doesn't need to travel far, it's less exposed to potential security breaches. Moreover, localized data storage means that sensitive information remains within the confines of the edge device itself, thereby enhancing security.
Lower bandwidth costs are another upside to using edge computing for ai applications. By reducing the volume of data that needs to be transmitted over networks, businesses can save substantially on their bandwidth expenses.
Autonomous Vehicles: A Case Study
Autonomous vehicles are an excellent example illustrating these benefits in action. These vehicles rely heavily on real-time decisions based on sensor inputs from their surroundings. The use case here involves leveraging edge ai solutions for processing this sensory data at lightning speed right within the vehicle itself (the "edge"). This allows autonomous cars to swiftly react to dynamic road conditions without any significant delay - a vital requirement for ensuring safe navigation.
Healthcare Industry Applications
Similarly, the healthcare industry has been increasingly adopting edge computing technologies for remote patient monitoring systems. Here's how it works:
- Medical sensors attached to patients collect vital health statistics.
- These stats are processed locally by an edge device.
- Only necessary information is sent back to healthcare providers.
This application not only aids in providing timely medical intervention but also ensures patient privacy as sensitive health data remains confined within local devices.
Energy Efficiency and Smart Cities
A notable benefit that often goes underappreciated is energy efficiency achieved through localized data processing at network edges. Less data transmission implies lower energy consumption which aligns well with global efforts towards creating more sustainable technologies.
Smart cities stand out as prime examples benefiting from this aspect of edge computing. Traffic management systems in smart cities employ localized processing units embedded within traffic lights or surveillance cameras at intersections; these units analyze real-time traffic conditions and adjust signal timings accordingly - all without needing extensive communication with central servers thus saving substantial energy resources over time.
With its myriad benefits and wide-ranging applications spanning various industries, it's no surprise that businesses worldwide are rapidly embracing edge AI solutions as part of their digital transformation strategies.
Deployment Models for Edge AI
Cloud-Edge Hybrid Model
The cloud-edge hybrid model combines the best of both worlds. It leverages the computational power and storage capabilities of cloud data centers while also utilizing the low latency and real-time processing abilities of edge devices. In this deployment model, machine learning models are trained in the cloud, then pushed to edge devices for inference.
- For instance, a company might train deep learning models on large datasets in the cloud, where resources are plentiful. Once trained, these models can be deployed on edge devices like smartphones or IoT sensors.
- This approach offers significant advantages in terms of scalability and flexibility. However, it also presents challenges related to security and connectivity. Data must be securely transmitted between the cloud and edge devices, which requires robust encryption protocols.
Fully Distributed Model
In contrast to the hybrid approach, a fully distributed model deploys AI models directly on edge devices without any reliance on central servers or cloud data centers.
- This deployment strategy is ideal for large-scale applications that require real-time decision-making at multiple points across a network.
- For example, an autonomous vehicle network would benefit from a fully distributed model as each car needs to make split-second decisions based on local conditions.
- While this approach offers superior latency and privacy benefits over other models, it poses unique challenges in terms of managing and updating machine learning models across numerous devices.
Centralized Model
The centralized model is often suitable for small-scale applications. In this setup, all AI processes take place within a single central server or data center.
- This deployment strategy simplifies management as all AI processes are handled centrally.
- However, it suffers from higher latency compared to other approaches due to reliance on transmitting data back-and-forth between edge devices and central servers.
Role of Micro-Data Centers
Micro-data centers play an essential role in deploying Edge AI applications by bringing computation closer to end-users.
- These miniaturized versions of traditional data centers house computing resources at or near the location where they're needed most.
- They help reduce latency by eliminating long-distance data transmission while providing localized storage capacity for machine learning models.
Deployment Challenges
Deploying Edge AI isn't without its challenges:
- Security: Protecting sensitive information becomes more complex when data is distributed across numerous locations.
- Connectivity: Reliable communication between different components is crucial but can be challenging due to network instability or bandwidth limitations.
- Scalability: Managing updates across multiple nodes can become cumbersome as scale increases.
Despite these hurdles, choosing the right deployment model can significantly impact cost-effectiveness by optimizing resource use based on specific application requirements.
Remember that no one-size-fits-all solution exists when selecting a deployment model for your Edge AI application; instead consider factors such as scale, latency requirements, security concerns before making your decision.
Edge AI's Role in Retail Industry
Personalized Customer Experience
Edge AI applications have revolutionized the retail industry by offering personalized customer experiences. One such application is smart shelves. These are IoT devices that use a combination of sensors and software to monitor inventory levels in real-time. They can alert store employees when stock levels are low or when items are misplaced, reducing the amount of time spent on manual inventory checks.
Moreover, smart shelves provide a unique shopping experience for customers. Using data collected from customer behavior analysis, retailers can offer targeted advertising based on individual preferences. This not only enhances the end-user experience but also increases sales for the business.
Real-Time Inventory Management
Managing inventory is a critical aspect of any retail business. With edge AI applications and IoT devices, businesses now have access to real-time data about their stock levels. This allows them to make timely decisions regarding replenishment and avoid overstocking or understocking issues.
In addition to improving response times, these systems also reduce the need for large-scale data storage as most computations happen at the edge - near where data is generated. This results in improved performance and reduced latency compared to traditional cloud-based services.
Improved Store Operations
Predictive maintenance powered by edge AI has been instrumental in improving store operations. By analyzing multi-dimensional sensor data from various equipment, these systems can predict potential malfunctions before they occur, allowing businesses to schedule maintenance activities proactively.
For example, refrigeration units used in grocery stores often require regular servicing to ensure optimal performance. An unexpected breakdown could lead to significant losses due to spoiled food items. However, with predictive maintenance powered by edge AI applications, such issues can be avoided.
Automated Checkout Systems
Edge computing technology has made it possible for retailers to implement automated checkout systems in their stores. These systems use computer vision algorithms and machine learning models trained on vast amounts of data captured at the edge (in-store). Customers simply walk into a store, pick up what they need and leave without having to wait in line at a cashier counter - an example being Amazon Go stores.
Automated checkout systems not only offer convenience for customers but also free up staff members who would otherwise be tied up at cash registers—allowing them more time for other tasks like assisting customers or restocking shelves.
In-Store Navigation Assistance
Augmented reality (AR) is another area where edge AI has shown immense potential in enhancing retail services. AR-powered navigation assistance helps shoppers find items quickly within a wide range of products available in large departmental stores or supermarkets.
The system uses AR markers placed throughout the store which interact with an app on the shopper's phone providing directions straight to desired products—saving customers' time and making shopping more convenient.
To sum up each point:
- Smart Shelves: Personalize customer experiences through targeted advertising
- Real-Time Inventory Management: Improve decision-making processes by providing real-time stock level information
- Predictive Maintenance: Enhance overall store operations by predicting equipment malfunctions
- Automated Checkout Systems: Provide seamless shopping experiences while freeing up staff members
- AR-Powered Navigation Assistance: Assist shoppers find items quickly within a wide range of products
The above points underscore how Edge AI plays an integral role within today’s competitive retail landscape—driving operational efficiency while delivering superior customer service.
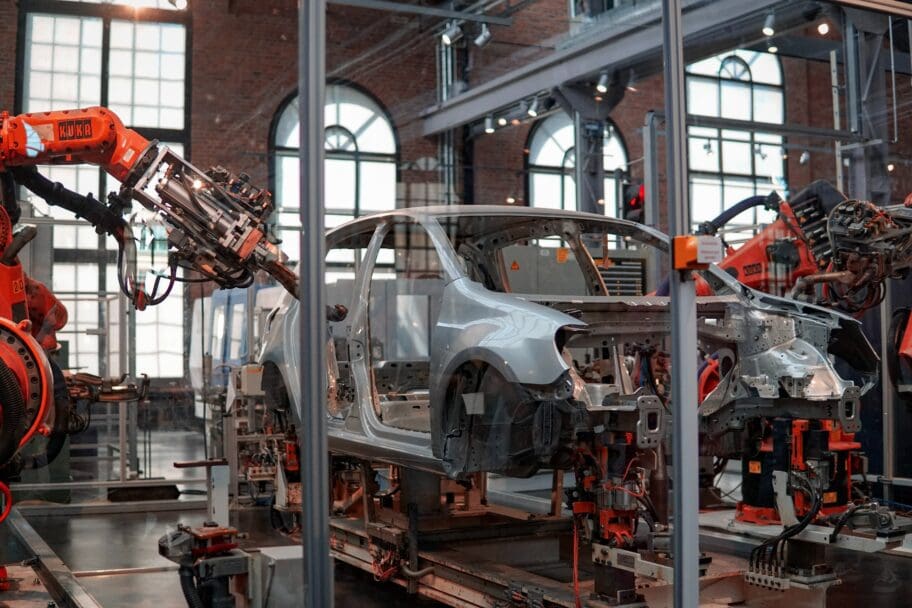
Manufacturing Industry Transformed by Edge AI
Predictive Maintenance: Reducing Downtime & Repair Costs
The manufacturing industry is witnessing a paradigm shift with the advent of edge AI applications. One of the most impactful applications lies in predictive maintenance. By harnessing machine learning algorithms and analytics, industries can anticipate potential machine failures and schedule repairs proactively. This approach significantly reduces downtime and repair costs.
For instance, consider an autonomous vehicle production line where each machine plays a crucial role. A sudden breakdown could halt production, leading to significant losses. But with predictive maintenance powered by edge AI, the system identifies patterns indicating potential issues before they occur. It might be an unusual spike in GPU usage or subtle variations in data processing times.
Edge AI performs these tasks on end devices like connected devices or smart devices installed on the machines themselves rather than relying on a data center. This local processing dramatically speeds up response times, ensuring that any impending faults are detected as early as possible.
Quality Control: Real-time Defect Detection
Another transformative edge AI application in manufacturing is quality control through real-time defect detection. Leveraging computer vision applications, manufacturers can now identify defects instantly during production stages.
In traditional methods, quality control relied heavily on manual inspections which were time-consuming and prone to human errors. However, modern camera technology combined with edge computing has revolutionized this process.
Smart cameras equipped with computer vision algorithms analyze images of products on the assembly line in real time. They compare these images against predefined standards to detect any deviations or defects instantly. The defective products are then removed from the assembly line automatically for further inspection or disposal.
This instant defect detection not only ensures high-quality products but also saves resources that would otherwise be wasted producing faulty goods.
Streamlined Supply Chain Management
IoT sensors have become ubiquitous in today's industries due to their ability to collect vast amounts of data from various points throughout the supply chain management process. When coupled with edge AI, these sensors can provide valuable insights into optimizing operations and reducing inefficiencies.
Consider a scenario where IoT sensors are tracking inventory levels across multiple warehouses spread across different locations:
- The sensor at warehouse A records low stock levels for a particular item.
- This data is processed locally using edge AI without needing to send it back to a central data center.
- Based upon past demand patterns and current market trends (also stored locally), the system predicts an imminent shortage at warehouse A.
- Simultaneously, excess stock of the same item is identified at warehouse B through similar processes.
- The system then initiates a transfer from B to A well before any actual shortage occurs at A.
This example illustrates how edge AI applications can streamline supply chain management resulting in reduced operational costs and improved customer satisfaction.
Smart Factories: Automation Powered by 5G & IoT
The concept of smart factories isn't new; however, their true potential is being realized now thanks to advancements in 5G technology and IoT devices powered by edge AI.
These factories leverage automation extensively for various operations ranging from raw material handling to final product packaging:
- Autonomous vehicles transport materials within premises based upon instructions received from localized data processing units.
- Robotic arms assemble components precisely according to design specifications fed into them via smart devices.
- Quality checks are performed continuously using computer vision enabled cameras detecting even minute defects promptly.
Future Trends in Edge AI
5G and Edge AI: A Powerful Combination
The integration of 5G technology into edge AI applications is a trend that's expected to skyrocket adoption rates. The high-speed data transmission offered by 5G networks perfectly complements the real-time analytics capabilities of edge computing devices. These devices, equipped with machine learning tasks, can process vast amounts of data streams at lightning speed, opening up new opportunities for various sectors.
For instance, in healthcare, patient monitoring systems could leverage this combination to provide real-time health status updates to medical professionals. In agriculture, smart irrigation systems could use real-time weather data to optimize water usage. Such practical applications not only illustrate the potential of edge AI but also justify the growing demand for it.
Expanding Use Cases Across Sectors
Edge AI's increasing use cases span across different sectors - from healthcare and agriculture to manufacturing and retail. Its ability to handle complex computing tasks like vision applications and natural language processing makes it an ideal solution for industries dealing with large volumes of time-sensitive data.
In healthcare, artificial intelligence integrated into edge computing devices can revolutionize patient monitoring by providing real-time health updates. Similarly, in agriculture, smart farming solutions using edge AI can optimize resource usage based on real-time climate conditions.
Energy Efficiency: A Key Development Goal
As we increasingly rely on cloud computing for executing machine learning tasks and managing large volumes of data streams, energy efficiency becomes a significant concern. The advances in edge AI are addressing these challenges by shifting some intelligence from the cloud to local devices (internet of things), thereby reducing energy consumption.
For example, instead of sending all raw data from a security camera (vision application) to the cloud for processing, an edge device could analyze the video feed locally and only send relevant clips or alerts to the cloud when necessary.
Investment Surge in R&D
With its promising capabilities and broadening application scope, there's no surprise that investment into research & development within this sector is on an upward trajectory. Companies are pouring resources into developing more advanced and efficient edge computing devices capable of handling complex machine learning tasks while consuming less power.
Privacy & Security: Top Priorities
As more sensitive information gets processed at the 'edge', privacy & security concerns naturally rise. This has led companies investing in Edge AI technologies to place greater emphasis on these aspects during development phases.
Sector |
Potential Application |
---|---|
Healthcare |
Real-Time Patient Monitoring |
Agriculture |
Smart Irrigation Systems |
Manufacturing |
Predictive Maintenance |
Retail |
Personalized Customer Experience |
This table illustrates just a few examples where Edge AI applications can make significant impacts across various sectors.
- Integration with 5G technology boosts adoption rates.
- Increasing use cases across various sectors.
- Rising demand due to growing need for real time analytics.
- Development towards more energy-efficient solutions.
- Increase investment into research & development within this sector.
- Greater emphasis placed upon privacy & security concerns.
Wrapping Up Edge AI for SMB Owners
Edge AI is revolutionizing the way small and medium-sized businesses operate, particularly in the retail and manufacturing industries. This technology offers numerous benefits such as real-time data processing, enhanced privacy, reduced latency, and lower bandwidth requirements. It's clear that Edge AI is not just a fleeting trend but an integral part of future technological advancements.
As a business owner, you can leverage these benefits to streamline operations, improve customer service, increase efficiency, and ultimately drive growth. Don't get left behind; start exploring how Edge AI can be integrated into your business model today. Remember that staying ahead of the curve often means embracing change and new technologies.
FAQs
What is Edge AI?
Edge AI is a technology that allows artificial intelligence algorithms to process data locally on a hardware device rather than in a centralized cloud or server.
How does Edge AI benefit my business?
Edge AI provides several advantages including faster decision-making due to real-time data processing, increased privacy as sensitive data remains on-device, reduced latency for improved user experience and less reliance on continuous internet connectivity.
Is Edge AI expensive to implement?
The cost of implementing Edge AI varies depending on your specific needs and existing infrastructure. However, it's important to consider the long-term savings it could bring through improved efficiency and performance.
Can I use Edge AI in my retail store?
Absolutely! Retail stores can benefit greatly from Edge AI by using it for tasks like inventory management, customer behavior analysis or automated checkouts.
How does the manufacturing industry benefit from Edge AI?
In manufacturing settings, edge computing can monitor equipment health in real time for predictive maintenance purposes. It also optimizes production processes by analyzing large amounts of data quickly on-site.
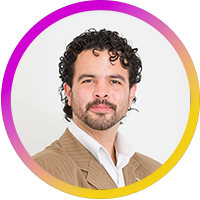
Article by
Titus Mulquiney
Hi, I'm Titus, an AI fanatic, automation expert, application designer and founder of Octavius AI. My mission is to help people like you automate your business to save costs and supercharge business growth!